Table of Contents
While tempting, don’t turn your CX initiative into a home-baked DIY artificial intelligence project. The stakes are too high, the technology is too complex and the people who will suffer most are your most precious assets: your customers.
Yes, you want to bend the curve of OpEx. Yes, your corporate finance department has frozen spending for the next year, so DIY seems reasonable. Yes, your hottest developers promise to build a game-changing customer-support platform.
Don’t fall for the seduction. We’ve engaged more than 100 companies that have gone down the path of DIY for an automated Conversational AI solution.
It has worked out exactly zero times.
With generative AI upon us, the urge to Do-It-Yourself is stronger than ever. Our seminal guide, DIY vs. Managed Service: Choosing the Right Approach for AI Implementation, can help you assess your choices.
But first, read on to see why DIY is almost always a mistake.
The DIY Pitfalls
When it comes to implementing a Conversational AI solution to fuel the customer-care strategy, companies are challenged with the ‘buy, build or partner’ problem.
Here are the top three reasons we see from companies considering DIY:
- Generative AI makes DIY development easier (False).
- IT leaders can assert control, save Opex costs, and own their tech stack (True, except for the savings. Factoring customer LTV and unexpected add-on costs, we’ve never seen this work. Ever).
- Low- or no-code options like Google are being bundled into legacy systems platforms (Integration takes years longer than forecasted nearly every time).
While the trend toward in-house development is understandable, stakeholders must recognize the many hidden costs and risks associated with this approach. Our experience observing more than 100 in-house DIY implementations and talking to the technology leaders is that hidden costs typically run 50-75% higher than original estimates.
DIY vs. Managed Service Explained in Two Minutes
Just the technical expertise of application development and API integrations are not enough to satisfy the requirements of setting up an IVA. Scientific expertise in Automated Speech Recognition, Natural Language Processing and machine learning is necessary to maintain the intelligence of a solution. Technologists and scientists with extensive experience in building speech recognition models, language and intent models and machine learning algorithms are an integral part of a successful implementation. In fact, to use any tool successfully, an application needs to be built that’s tied to your company’s complex business rules. This level of customization requires a team of speech, NLP and IT specialists—all of which are in high demand.
Interactions Does the Heavy Lifting, but we can Leaverage Your Expertise
Are You Looking for a Quick Fix or a Sustainable Solution?
Although the traditional build vs. buy scenario has been around for years, implementing an advanced technology such as Conversational AI into an existing infrastructure and designing it in a way that delivers positive experiences every time, introduces a new set of challenges.
Missed or underestimated costs fall into three categories:
1. Larger than expected investment in resources and talent
Developing Conversational AI technology requires substantial investments in resources and talent such as linguists, data scientists, software engineers, UX designers, and domain specialists. Knowing that your company has the resources to successfully pull off DIY AI is critical. Only 1 in 10 organizations are able to get 75% or more of their AI model prototypes into production, according to the Gartner AI in Organizations Survey.
One example: a company came to Interactions after attempting to build an AI solution using Google. Their in-house team of four spent six months developing just one use case. But our discovery revealed a need for 30 use cases. If you play out that math, it looks like this:
Assuming an average annual salary of $150,000 per person, staff costs alone are astronomical, not to mention the glacial time to complete the project, which takes us to our next risk: development time.
Key Takeaways
The expertise required for truly scalable Conversational AI:
- The need for a deep understanding of speech recognition, NLU, and dialogue science
- The advantages of having dedicated teams to build voice assistants tailored to specific customer journeys
- The importance of close collaboration across all layers of the Conversational AI stack
2. Lengthy development time and delayed deployment
Developing Conversational AI applications is a continuous process that involves multiple iterations and feedback loops. Companies need to collect and analyze large amounts of data to train and optimize the models and test them for accuracy, performance, and usability. Applications must also be compatible with different platforms, devices, and languages—steps that can take months or even years to complete.
Another company that came to Interactions had a legacy DTMF telecommunications system but decided to use Google’s contact center AI to build a platform in-house. A team of five people spent 12 months in development with no success, then hired Google to complete the project. They abandoned the project after another 18 months. The result was an AI platform that didn’t come close to the performance of their original 20-year-old system. This is not an anomaly; we have seen similar situations dozens of times.
Key Takeaways
The drawbacks of in-house Conversational AI development:
- The high cost and difficulty in building comprehensive capabilities
- The challenges in recruiting and retaining specialized talent
3. Significant maintenance and continuous improvement
Conversational AI requires constant monitoring and fine-tuning to ensure data relevance and accuracy to adapt to changing customer expectations, preferences, behaviors, and feedback. Once an in-house solution is built and deployed, companies need a product owner and technical staff to oversee maintenance and program new use cases.
A larger regional utility company considering DIY came to Interactions for IVA support because they didn’t want to take on the cost of additional staff to maintain the product and didn’t have a manager to own success metrics for the platform. Without sufficient post-launch staffing or leadership, DIY projects will fail to thrive or survive.
Key Takeaways
The challenge of DIY Conversational AI:
- Inability to effectively handle real customer interactions
- Limited options for managing and controlling risks during live deployment
- General-purpose Conversational AI platforms lacking optimization for phone support and customer service conversations
AI implementations are NOT like typical IT projects.
Conversational AI is not one technology. It is a synchronized array of many underlying technologies, such as ASR, NLP, Dialog Management, and Machine Learning.
Conversational AI needs many experts. Skilled teams from many disciplines are required to build, train, implement, and maintain; a few app developers cannot build AI solutions.
Advanced Conversational AI needs design expertise. AI deployments can seem clunky and robotic without design expertise, leading to an unpleasant customer experience.
AI systems need constant training and tuning. The typical “set it and forget it” deployment approach doesn’t work for AI.
Deep domain knowledge is essential. Building a Conversational AI solution for customer care requires deep domain knowledge of the contact center and the customer experience industry.
Weighing the Risks
In addition to hidden costs, there are other risks associated with DIY Conversational AI development:
Inability to keep up with rapid advancements in AI technology
AI is part of every modern roadmap in the contact center ecosystem. Transformative technology advancements and techniques are emerging more rapidly – at a dizzying speed – as generative AI implementations accelerate. Developing Conversational AI in-house requires continuous monitoring, research, and adaptation to stay abreast of these advancements, which can be challenging for many businesses or IT teams who must move on to the next big project.
Companies need to ask themselves a variety of questions before choosing an implementation strategy, including:
- Do we have the right AI talent in house?
- If not, can we hire the right people?
- Do we have an AI strategy?
- How does a Conversational AI solution fit into that? Is it related to our core competency?
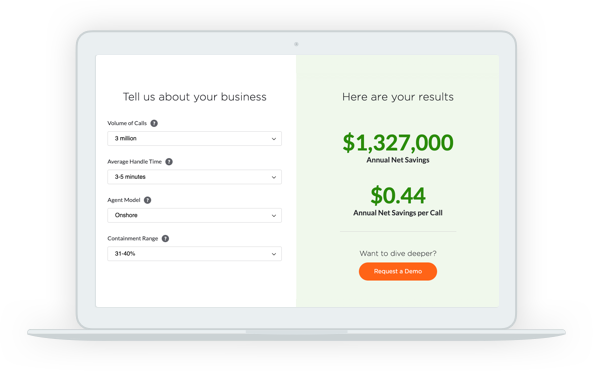
See your savings
Potential for subpar customer experiences
Creating a high-quality Conversational AI experience requires extensive data collection, model training, and fine-tuning. Industry experts at managed-service providers (MSPs) have access to large and diverse datasets, allowing them to train their models more effectively and provide accurate and natural interactions. In-house development often lacks access to such extensive datasets which can lead to bad customer experiences and failed expectations.
Speaking of bad customer experiences, there’s an adage that says “you only get one chance at a good first impression.” Inexperienced DIY implementers and immature Conversational AI systems may deliver poor initial performance at the time of launch. Even if the experience is improved through tuning over the first few months, you may sour or lose both internal and external clients during the early days.
Security and compliance concerns
Conversational AI systems are sophisticated enough to handle sensitive customer data in fast, friction-free ways, ensuring security and compliance standards are achieved. Developing an in-house solution to meet these standards is a tall order. It requires meticulous attention to data privacy, security protocols, and compliance with regulations such as GDPR and CCPA. Company leaders must consider whether they want to take on these additional risks.
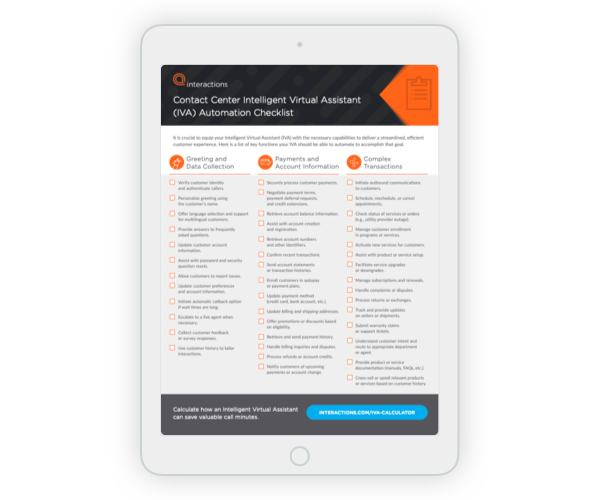
Considering Conversational AI for your contact center?
DIY strategies are not ideal for enterprise applications.
Enterprises have complex considerations including:
- the number of use cases,
- the channels of engagement,
- the rate of change in the products, services, and customers of the enterprise organization,
- range of demographics in their customer base, and
- and for those with a global operaton, the languages, and regions
All of these factors call for a large supporting team of specialists that can remain abreast of rapidly changing technology and capture best practices in the many areas required to take on a DIY solution.
Not their primary focus: Amazon Lex, Google CCAI, Nuance, and other DIY AI technology providers have plenty of other products and priorities to focus on.
Offer disjointed pieces of technology: Conversational AI is not a single technology but a synchronized array of underlying technologies. Experts are needed at initial implementation and to stay abreast with all aspects of AI, conversational design, generative aspects, and model training to name a few. DIY also requires an expert architect to meld all the components that lead to a good customer experience that delivers operational savings. For DIYers, this can distract from the core expertise needed to remain on top of the game for their core business.
More suited to test the waters: The DIY platforms lack the level of maturity to train intent models to fit complex customer care applications. They provide a starting point with something seemingly straightforward. But to get from an initial trial use case to the sophisticated use cases needed for the business requires considerable investment and often stretches the capabilities of these platforms.
Present data security and privacy concerns: Does the platform meet compliance and security certification needs? The burden of compliance responsibility will fall on the Enterprise to monitor and ensure compliance. Another area requiring not only collaboration of the DIY platform vendor, but identification of specialized internal expertise, that may stretch the scope of existing security teams.
A better solution is a close partnership with a provider who allows a high degree of control and configurability, and collaboration may yield more effective, timely, and flexible solutions
DIY Alternatives
Given the costs and risks of DIY Conversational AI, it makes sense to partner with industry experts who provide best-in-class solutions matched to your business needs. Working with an MSP like Interactions offers several benefits:
Access to cutting-edge technology and expertise
Industry experts in Conversational AI have extensive knowledge and experience developing sophisticated solutions. Combined teams have invested 100 of years of research and development to stay at the forefront of technological advancements. They have managed dozens of complex, customized environments. The importance of their expertise for building successful platforms, navigating pitfalls and delivering on time and on budget cannot be overstated.
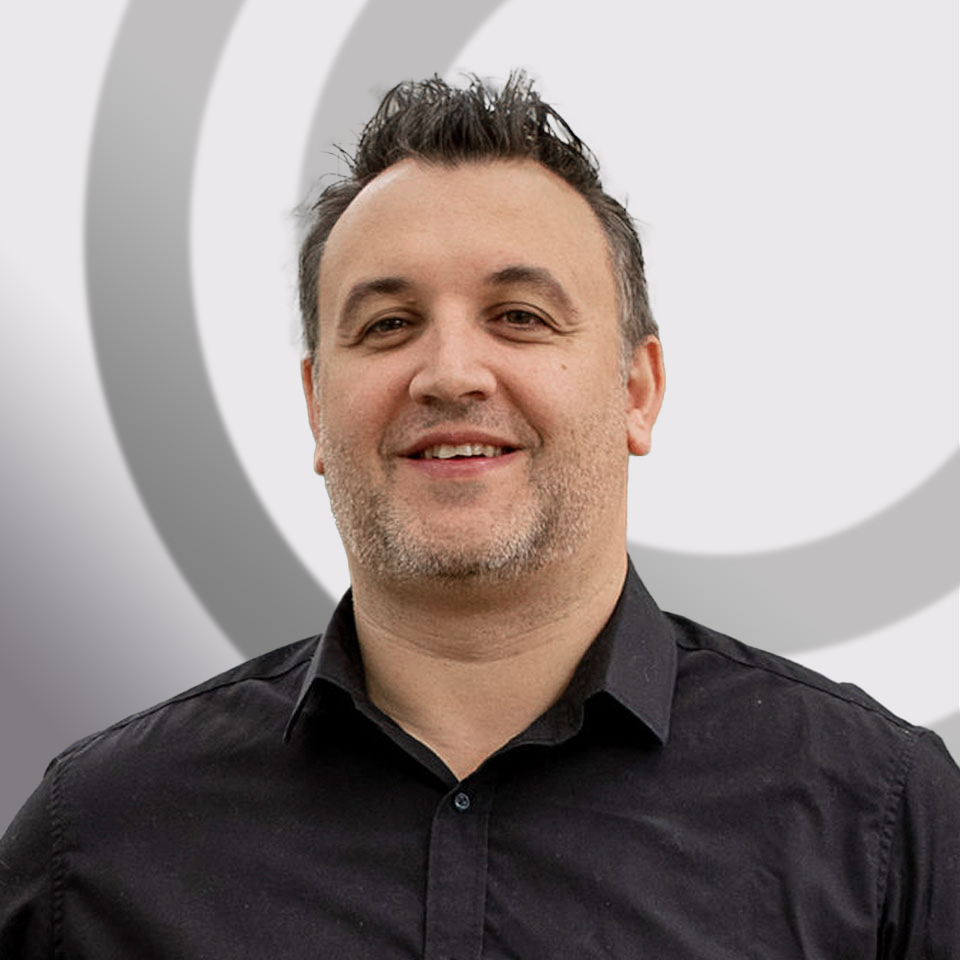
Faster implementation and reduced time-to-market
By leveraging AI industry specialists’ expertise and ready-made, customizable templates, components, and frameworks, businesses can significantly accelerate the implementation process.
Ongoing support and improvements to stay ahead of the competition
MSPs also provide dedicated support teams that ensure the smooth operation of Conversational AI systems. They proactively address issues, offer ongoing maintenance, and continuously improve solutions based on customer feedback and emerging trends, something DIY platforms cannot do.
Success Stories: Driving Innovation Together
Many brands have recognized the need to partner with a MSP like Interactions to deploy effective, scalable Conversational AI solutions. Here are two examples of successful AI deployments from our utilities team:
When utility provider Evergy acquired Westar Energy, an existing client of Interactions, they implemented AI capabilities across their entire business. The move from their legacy system to Interactions’ IVA resulted in:
- 75% increase in payment success rate
- 5% improvement in CX scores
- 25% or more improvement in JD Power scores
Texas utility company TXU Energy partnered with Interactions to improve customer experience, gain a competitive edge, and reduce operating expenses. Interactions replaced a DIY speech system that was unable to keep up with call volume demands of more than a million calls per month. After implementing Interactions’ self-service application, TXU saw the following:
- 24% call center volume drop: $2.5m annual savings
- $750,000 IT savings; $200,000 in cost avoidance
- 40 days: achievement of their payback goals
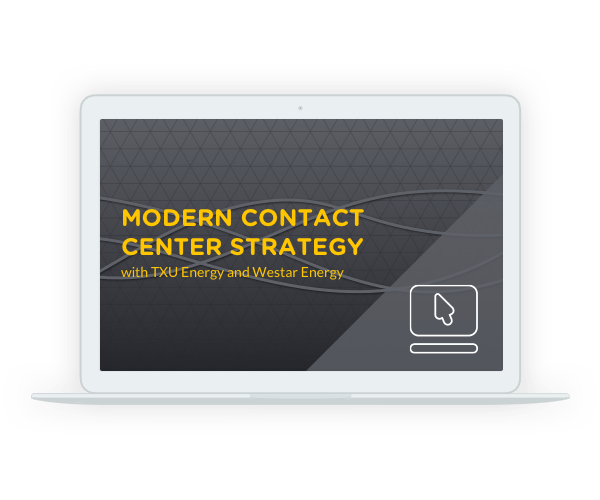
Listen to the Experts
Your Path to Conversational AI Success
Conversational AI is one of the most effective ways to improve customer experience, increase workforce productivity, decrease operational costs, and drive revenue. But today’s market requires that CIOs and IT leaders accelerate time to value for digital investments.
If you’re considering DIY development, you should carefully weigh the costs and risks against the advantages of an MSP. Can your company afford to wait years and invest capital in a solution that may not give you the necessary use cases?
Key Takeaways
The benefits of partnering with expert-managed services:
- Access to world-class engineering and research talent
- Customized Conversational AI platforms designed specifically for customer service
- Proven track record in outperforming general-purpose Conversational AI solutions
Interactions offers a more affordable, fully managed Conversational IVA solution. Our platform is also vendor agnostic, which protects your investments in legacy systems and any future investments. These are just some of the reasons the world’s largest enterprises trust our Conversational AI products.
Want to take a deeper dive? Download our whitepaper entitled “DIY vs. Managed Service: Choosing the Right Approach for AI Implementation”.